- Home
- Microsoft Certifications
- AI-900 Microsoft Azure AI Fundamentals Dumps
Pass Microsoft Azure AI AI-900 Exam in First Attempt Guaranteed!
Get 100% Latest Exam Questions, Accurate & Verified Answers to Pass the Actual Exam!
30 Days Free Updates, Instant Download!
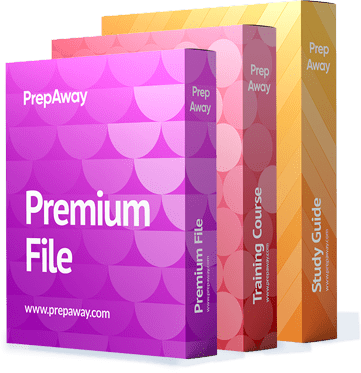
AI-900 Premium Bundle
- Premium File 298 Questions & Answers. Last update: Oct 22, 2024
- Training Course 85 Video Lectures
- Study Guide 391 Pages
Last Week Results!

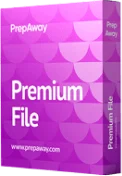
Includes question types found on the actual exam such as drag and drop, simulation, type-in and fill-in-the-blank.
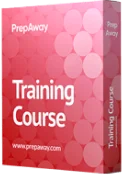
Based on real-life scenarios similar to those encountered in the exam, allowing you to learn by working with real equipment.
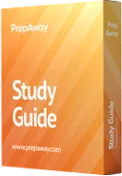
Developed by IT experts who have passed the exam in the past. Covers in-depth knowledge required for exam preparation.
All Microsoft Azure AI AI-900 certification exam dumps, study guide, training courses are Prepared by industry experts. PrepAway's ETE files povide the AI-900 Microsoft Azure AI Fundamentals practice test questions and answers & exam dumps, study guide and training courses help you study and pass hassle-free!
Describe fundamental principles of machine learning on Azure
20. Lab - Building a Regression Machine Learning Pipeline - Complete Pipeline
Now that the pipeline is complete, I can move on to the missing data module. I can then right-click and visualise the clean data set. So over here, you can see we don't have that column in place. We now only have 25 columns, and let me go on to the horsepower of that particular feature. You can see we have zero missing values, so even the number of rows has decreased. So now we have a clean data set in place, and the remaining things remain the same. So we need to go ahead and train the model. So over here, let me go ahead and choose the train model.
Drag it over here, and we'll close this for now. Then we have to go ahead and split our data set. So let's go ahead and split the data. So, 0.7, I'll drag it over here again, and please allow me to connect the missing data to the split data. Then over here, let me go ahead and take the split data and use it to train our model. Now, in terms of the machine learning algorithm, I'm going to go ahead and choose linear regression since we're doing regression over here. So let me go ahead and just leave everything as it is.
Let me go ahead and connect it to the train model. Now that we're in the model, let me go ahead and choose the column. So I'd like to predict what the price column will be. I'll go ahead and hit save next. Let's go ahead and score the model. So this will be based on the training and also the test data, and then we will finally go ahead and evaluate our model. So drag it over here again, connected right, so we have everything in place, and let's go ahead and hit on submit again. I'll choose the same existing experiment, and let's go ahead and click on submit. Alright, so again, this will take time. Let's come back once this is complete to go ahead and evaluate the results.
21. Lab - Building a Regression Machine Learning Pipeline – Results
Now is an awesome time. When I come back, I can see that my pipeline execution is complete. So our model has been evaluated. Again, we can go ahead and right-click on "evaluate model," and we can go ahead and visualise the evaluation results. Now, the type of results that are shown to you will be different over here. So it will not be the same as the results we have seen in the classification learning algorithms. So, depending on the algorithm, the type of algorithm that you choose, you will get different model results. So over here we have results such as the mean absolute error, the root mean squared error, etc.
So these are the results that we have in place. So, just to quickly go ahead and explain a couple of them, So the mean absolute error is the expected value of the absolute value of the difference between the target and the prediction. And then we also have the root mean squared value. So again, just to give you kind of an idea, remember that when it comes to linear regression and trying to find the correlation between the data values, it goes ahead and basically creates a light. I'm just giving a very simple example based on the data points.
Now, when it tries to make a prediction, there might be data values on this side of the line and data values on the other side of the line. So over here, the distance between the data point, the prediction, and the line is kind of an error because it's a deviation from what the prediction should be given by the model itself. Now, since this prediction can be on either side of the line and since you can't have negative values, that's why it goes in and creates a square of these values, and it basically gives you this as the error index. So again, these are all the results that you get with a regression-based algorithm. In this case, you've chosen linear regression, right? So this marks the end of this chapter. So we have gone ahead and seen how to use a regression algorithm when it comes to machine learning.
22. Feature Engineering
Hi, and welcome back. Now in this chapter, I just want to go through two important concepts when it comes to features. So remember what we saw earlier in your data set for training a machine learning model; you'll go ahead and give data. So these are all of your features, and then you want to go ahead and predict the label, which is your income.
So we have seen an example when it comes to the classification model. Now, sometimes, if you have your data in place, you can go ahead and use that data to go ahead and generate new features. This is known as "feature engineering" if you feel it in your raw data. So over here, I'm giving an example of an existing data set that we have for your machine learning. But you might have your own data in place.
So this is your raw data. And let's say you want to go ahead and create a data set out of that. Now you might already have some features in place, but maybe from that raw data you can go ahead and create a new feature column that could be a combination of the raw data that you have. It could be that that column could actually go ahead and increase the predictive power of the machine learning algorithm and the model. If you feel this might be the case, then you can actually go ahead and create that new feature. This is known as "feature engineering." Then you have something known as feature selection.
Now, sometimes, from your list of features, it might be the case that not all features are required when it comes to training your machine learning model. So, for example, if I go ahead and scroll down, let's say I have this data set in place, let's say this is my label, and initially I believe these should be my features. Do you believe that having the first and last name be a determinant of how the income range would actually be beneficial?
No. Right. So there is no point in having these columns in place. You don't need the first and last names. So over here, you can actually go ahead and remove these columns from your data set. This is known as feature selection. Over here, you can go ahead and choose the features of the educational level and the age. So in this chapter, we're going to go through those two important concepts of feature engineering and feature selection.
23. Automated Machine Learning
Hi, and welcome back. Now in this chapter, I want to go through automated machine learning. So this is a feature that is available within Azure machine learning. So the entire purpose of this feature is to automate the time-consuming process that is involved in machine learning development itself.
So, for example, if a data scientist wants to go out and train a model, they may need to try out different machine learning algorithms before deciding which one is best for training their model. This is determined by the model's output following the training process. So in order to go ahead and save time in trying to find out which algorithm can train the model the best, you can actually go ahead and make use of automated machine learning. So the normal process of checking the accuracy of models against different machine learning algorithms is a time-consuming task. So this can be automated with the help of automated machine learning. So this can be used for both the classification and regression models. So let's go ahead and jump onto Azure ML machine learning and see how we can work with automated machine learning.
So here we are at the designer. So please allow me to return home. Now over here, we can go ahead and choose automated ML and click on "Start Now." Now we can go ahead and click on "new automated machine learning run." Now over here we can go ahead and choose our data set. But what we can do is that, again, we can go ahead and choose from a data set that exists for your machine learning. So I can go ahead and choose the option from Open Data Sets.
Now over here, I'm going to go ahead and choose the sample diabetes data set. If you want to go in and learn more about the data set, you can actually go ahead and click on the "Learn More" link over here. Now we're going to go into depth when it comes to automated machine learning from the perspective of the exam. You just have to go in and understand the purpose of automated machine learning. So let me go ahead and choose the sample diabetes data set. I'm going to go ahead and hit Next. Now we can go ahead and create a dataset out of the sample diabetes data set.
So I'll just give it a simple name. I'll go on to create now; let me go ahead and choose that data set and click on Next. Next, you need to go ahead and configure an experiment. So let me go ahead and create a new experiment. I'll just give a name now in terms of the target column. So I'm going to go ahead and choose the label column; that's why.
So again, the diabetes data set takes into account different aspects of a person and gives a diabetes range that is actually marked as the label column of Y. Again, I'm not going through the data set itself because, in this case, I decided to explain to you the purpose of automated machine learning. So let me go ahead and choose the label column. Now over here, again, we have to go ahead and choose a compute cluster since I already have one in hand. Let me go ahead and choose that and hit next. Now over here, it's going to go ahead and choose the classification category when it comes to machine learning. You can also go ahead and use regression, and you can use time series forecasting as well.
Now over here, if I go on to view additional configuration settings, over here there is a setting that explains the best model and also gives you the primary metric of accuracy. So over here, you can decide on what should be the primary metric when it comes to evaluating the models and also explain the best model. So this gives you more transparency when it comes to what automated machine learning is actually trying to do. So remember, it's going to go ahead and train the model based on the data set and the different algorithms that are actually available in machine learning. So let me go ahead and hit save, and then we go ahead and hit finish. So now it's going to go ahead and create a new automated machine-learning run. This entire process will actually take time; it could take hours. And that's because it's not going to go ahead and, in the background, create a pipeline, take the data set, take each machine learning algorithm, and then try to build or train a model. So let me just come back after half an hour and let's see the progress of this machine learning run.
Now, I've come back after an R, and the automated machine learning is still running. Now, if I go on to the models over here, So in the models, you can see that all of the algorithms are being tried out in the training of the model for each of the algorithms. You can go ahead and see the accuracy over here. So now what automated machine learning is doing is that it's actually going ahead, taking that data set, training models based on the different algorithms, and then giving you results in terms of accuracy. You can also go in and choose which model you want to use. So this entire thing of building the machine learning model via different algorithms is being automated by the automated machine learning service, right? So in this chapter, I'm going to mark this as an end. I just want to go ahead and show what the entire purpose of automated machine learning is.
24. Deleting your resources
Hi, and welcome back. So we've reached the end of this section after looking at machine learning labs in SEW. Now, if you've been following along to ensure that you don't bear any additional cost, make sure to go and delete the resources that you create. So if you've gone ahead and created the automatic machine learning pipeline, in my case, it's still running. So let me go ahead and press cancel to cancel this run of automated machine learning. Aside from that, be sure to visit your compute section. So if you've gone ahead and created an inference cluster, a Kubernetes service, go ahead and choose that and hit delete.
So this will go ahead and delete the inference clusters, go on to your compute clusters, and go ahead and delete your compute cluster from here itself. Once all these resources have been deleted, go on to Azure. So make sure to see all the resources that are part of that resource group. So for me, it's part of the Learning GRP resource group. So over here So we just went ahead and said, "Go ahead and delete the Kubernetes service," and it's done. But apart from that, you have to go ahead and ensure that you delete the container history, the machine learning workspace, the keyword, the storage account, and application insights. So go ahead and delete all of these resources since they are not required. So ensure that at the end of all of this, once you finish practising with elaborate, you go and delete the resources that are not required.
Microsoft Azure AI AI-900 practice test questions and answers, training course, study guide are uploaded in ETE Files format by real users. Study and Pass AI-900 Microsoft Azure AI Fundamentals certification exam dumps & practice test questions and answers are to help students.
Exam Comments * The most recent comment are on top
Purchase AI-900 Exam Training Products Individually
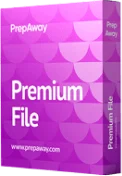
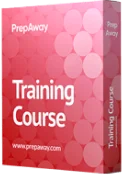
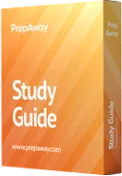
Why customers love us?
What do our customers say?
The resources provided for the Microsoft certification exam were exceptional. The exam dumps and video courses offered clear and concise explanations of each topic. I felt thoroughly prepared for the AI-900 test and passed with ease.
Studying for the Microsoft certification exam was a breeze with the comprehensive materials from this site. The detailed study guides and accurate exam dumps helped me understand every concept. I aced the AI-900 exam on my first try!
I was impressed with the quality of the AI-900 preparation materials for the Microsoft certification exam. The video courses were engaging, and the study guides covered all the essential topics. These resources made a significant difference in my study routine and overall performance. I went into the exam feeling confident and well-prepared.
The AI-900 materials for the Microsoft certification exam were invaluable. They provided detailed, concise explanations for each topic, helping me grasp the entire syllabus. After studying with these resources, I was able to tackle the final test questions confidently and successfully.
Thanks to the comprehensive study guides and video courses, I aced the AI-900 exam. The exam dumps were spot on and helped me understand the types of questions to expect. The certification exam was much less intimidating thanks to their excellent prep materials. So, I highly recommend their services for anyone preparing for this certification exam.
Achieving my Microsoft certification was a seamless experience. The detailed study guide and practice questions ensured I was fully prepared for AI-900. The customer support was responsive and helpful throughout my journey. Highly recommend their services for anyone preparing for their certification test.
I couldn't be happier with my certification results! The study materials were comprehensive and easy to understand, making my preparation for the AI-900 stress-free. Using these resources, I was able to pass my exam on the first attempt. They are a must-have for anyone serious about advancing their career.
The practice exams were incredibly helpful in familiarizing me with the actual test format. I felt confident and well-prepared going into my AI-900 certification exam. The support and guidance provided were top-notch. I couldn't have obtained my Microsoft certification without these amazing tools!
The materials provided for the AI-900 were comprehensive and very well-structured. The practice tests were particularly useful in building my confidence and understanding the exam format. After using these materials, I felt well-prepared and was able to solve all the questions on the final test with ease. Passing the certification exam was a huge relief! I feel much more competent in my role. Thank you!
The certification prep was excellent. The content was up-to-date and aligned perfectly with the exam requirements. I appreciated the clear explanations and real-world examples that made complex topics easier to grasp. I passed AI-900 successfully. It was a game-changer for my career in IT!