Pass Amazon AWS Certified Machine Learning - Specialty Exam in First Attempt Guaranteed!
Get 100% Latest Exam Questions, Accurate & Verified Answers to Pass the Actual Exam!
30 Days Free Updates, Instant Download!
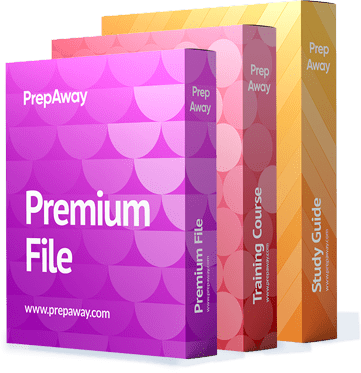
$39.99
AWS Certified Machine Learning - Specialty Premium Bundle
$69.98
$109.97
- Premium File 369 Questions & Answers. Last update: Jul 01, 2025
- Training Course 106 Video Lectures
- Study Guide 275 Pages
$109.97
$69.98
Last Week Results!

90
Customers Passed Amazon AWS Certified Machine Learning - Specialty Exam
Average Score In Actual Exam At Testing Centre
Questions came word for word from this dump
Get Unlimited Access to All Premium Files
Details
Purchase AWS Certified Machine Learning - Specialty Exam Training Products Individually
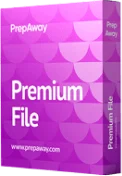
Premium File 369 Q&A
$65.99$59.99
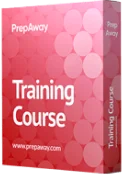
Training Course
106 Lectures
$27.49
$24.99
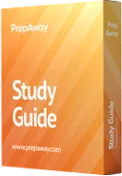
Study Guide
275 Pages
$27.49
$24.99
Why customers love us?
93%
Career Advancement Reports
92%
experienced career promotions, with an average salary increase of 53%
93%
mentioned that the mock exams were as beneficial as the real tests
97%
would recommend PrepAway to their colleagues