Pass Microsoft Data Science DP-100 Exam in First Attempt Guaranteed!
Get 100% Latest Exam Questions, Accurate & Verified Answers to Pass the Actual Exam!
30 Days Free Updates, Instant Download!
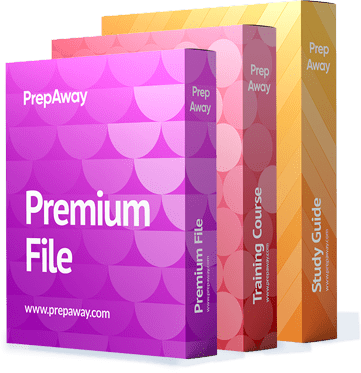
$39.99
DP-100 Premium Bundle
$69.98
$109.97
- Premium File 400 Questions & Answers. Last update: Jul 08, 2025
- Training Course 80 Video Lectures
- Study Guide 608 Pages
$109.97
$69.98
Last Week Results!

67
Customers Passed Microsoft DP-100 Exam
Average Score In Actual Exam At Testing Centre
Questions came word for word from this dump
Get Unlimited Access to All Premium Files
Details
Purchase DP-100 Exam Training Products Individually
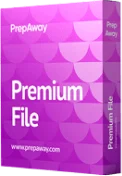
Premium File 400 Q&A
$65.99$59.99
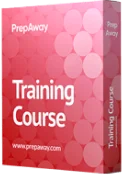
Training Course
80 Lectures
$27.49
$24.99
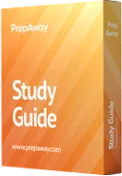
Study Guide
608 Pages
$27.49
$24.99
Why customers love us?
93%
Career Advancement Reports
92%
experienced career promotions, with an average salary increase of 53%
93%
mentioned that the mock exams were as beneficial as the real tests
97%
would recommend PrepAway to their colleagues
Million thx