Pass Microsoft Certified: Azure Data Scientist Associate Certification Exam in First Attempt Guaranteed!
Get 100% Latest Exam Questions, Accurate & Verified Answers to Pass the Actual Exam!
30 Days Free Updates, Instant Download!
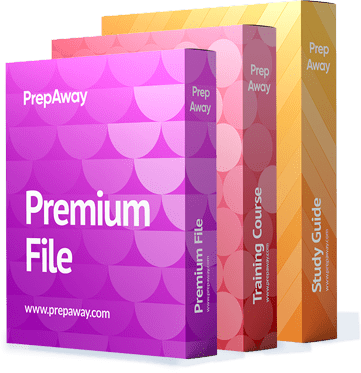
DP-100 Premium Bundle
- Premium File 527 Questions & Answers. Last update: Feb 16, 2025
- Training Course 80 Video Lectures
- Study Guide 608 Pages
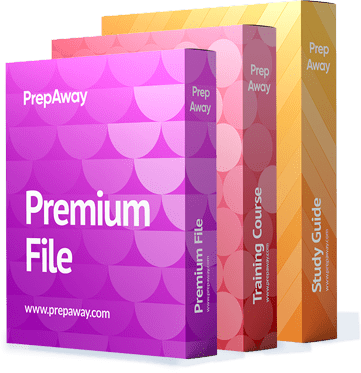
DP-100 Premium Bundle
- Premium File 527 Questions & Answers
Last update: Feb 16, 2025 - Training Course 80 Video Lectures
- Study Guide 608 Pages
Purchase Individually
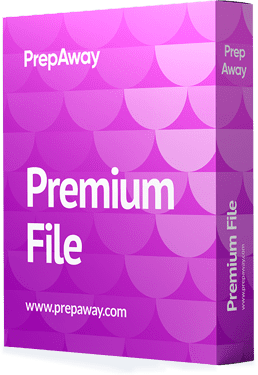
Premium File
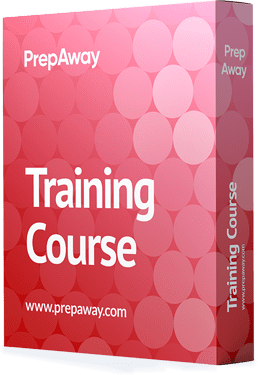
Training Course
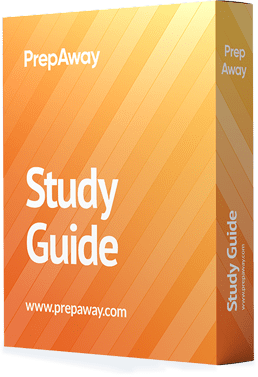
Study Guide
DP-100 Exam - Designing and Implementing a Data Science Solution on Azure
Download Free DP-100 Exam Questions |
---|
Microsoft Microsoft Certified: Azure Data Scientist Associate Certification Practice Test Questions and Answers, Microsoft Microsoft Certified: Azure Data Scientist Associate Certification Exam Dumps
All Microsoft Microsoft Certified: Azure Data Scientist Associate certification exam dumps, study guide, training courses are prepared by industry experts. Microsoft Microsoft Certified: Azure Data Scientist Associate certification practice test questions and answers, exam dumps, study guide and training courses help candidates to study and pass hassle-free!
Deploy Webservice
1. Azure ML Webservice - Prepare the experiment for webservice
Hello and welcome. Thus far, we have created many models using various types of algorithms. But what good are they if we can't use them? Almost all the tutorials teach you how to develop the model, but very few tell you how to use it by deploying it. There are other challenges as well. What if the model language is not supported or is difficult to deploy in the current architecture? Many times, environment setup can be tedious, and you may require various installations and configurations. You may even encounter many such challenges during the model's deployment using various other packages. However, Azure makes it very simple, so let's go to the Azure ML studio and see how we can deploy one of our models as a web service.
It's going to be pretty easy, and again, without writing a single line of code. Well, I have this experiment that predicts income based on the census. We created this model and successfully tested it with very high accuracy. In this experiment, I have first cleared the missing data by removing those rules, edited the metadata, and made some of the columns categorical as required. Then we also split the data set for training and testing, which is a 70/30 ratio. The algorithm we used is a two-class boosted decision tree. I have already run the experiment, so let's visualise the results. All right, all looks good, and we have very good accuracy as well as an excellent AUC. Let me close this. And the next step is to set up the experiment as a web service. Let's do that in the next lecture. Thank you so much, and see you soon.
2. Deploy Machine Learning Model As a Web Service
Hello and welcome to this lecture. Let's set up our web service. We have this experiment that has run successfully, so we go to the Setup WebService and click on Predictive Web Services. Azure ML takes care of creating an input based on the data set we have as well as creating a trained model that will predict the outcome. However, as you can see, the webservice output is the scored model. The next step is to run the predictive experiments so that any changes we make can be validated. So let's run the predictive experiment. All right. It has run successfully, and let's visualise the score model. Okay, it has all the features from the input data set that we selected, as well as the score level and score probabilities.
We can change that at this stage of the predictive experiment if we do not want all the features of the Score Model data set. Okay, we can add a select column and select only those that we need. Let's say we need only score levels and score probabilities. So we then need to add a select column module. Let's search for it. And there it is. Let me drag and drop it onto the canvas. We can also change the connections to the Web service output here, which is what we are going to do. And let me drop this connection between the score model and web service output. Let's bring the select column in between and make the right connections.
This one goes here, and this one goes right here. Let's now launch the column selector so that we can choose the two columns of "score label" and "score probability." All right, I selected these two columns and clicked OK. And let's run the predictive experiment again so that our changes take effect. Alright, it has run successfully; let's deploy it as a web service by clicking on the Deploy Web Service Option. Wow, our web service is now ready. We can either call it from some application or even call it from Excel. We will call this web service Excel, something that we are all familiar with. So tune in to the next lecture, where we will call this Web service and predict the result. Thank you so much, and see you soon. You.
3. Use the Web Service - Example of Excel
Hello and welcome. In today's lecture, we will cover how to consume our web service of adult census income predictions from Excel. Let me first explain the various things we see on this particular page. The new web service experience links you to the quick start. And if we go to the dashboard, it shows various parameters of how many requests have been made, what the average compute time was, the average job latency, and so on. We have just created this web service, so all these statistics are zero. All right, let's come back to the Quick Start. As you can see, we can either test or configure the endpoints, as well as use them. It also allows you to launch the web service in Excel.
I'll show you how to use it in Excel. But first, let me show you what each of these basic tabs has to offer. If we go to Configure, you can change the description and what kind of logging you can have for this web service. However, logging can only be enabled for paid workspaces. If the sample data is enabled, you can test the endpoints using the sample data. We will see that in just a bit. Let's click on "consume." It displays various information for us to consume this web service. All right. As you can see, we can do that using Excel as well as an application, and it shows various authentication information for the same, such as the primary key, secondary key, API link, as well as the sample code for C, Hash, Python, and R. We can also test this web service by going to the Test tab. as we have not enabled the sample data to test it. It shows us this message. Now let's enable it so that we don't have to type it all over.
All right, we now have some sample data prepopulated, so let's test the Request Response API. Okay, let me check the result. And there you go. It has the score level and the probabilities predicted for it. in case you want to use it in Excel. Let's go to Consume and click on Excel so we can download the Excel template and consume the web service in Excel. Alright, let me save this. It has finished downloading; let me open it. Now I have to enable editing so that I can use it. If you open it for the first time, it may ask you to enable the Azure ML web service. Okay, let me enable editing. And as you can see, it brings up this small dashboard on the right. Let's select our web service for adult sensors income. Okay, here I can configure the input source names and output destinations.
As I do not want to type the entire featureset, I am going to use sample data to do that for me as I click on it. It has populated some rows from the data set. Okay, as we only need one row, which can act as the input to this web service, I am going to delete all the other rows and select the header and one input row just like rows and seleSo let's click on "input" and click "OK." So, since we've chosen the header, we'll keep my data with the header checked.
Okay, next we need to set up where to display the output. I'm going to use this particular sale for the same and type it here. Okay, let's now click on "predict," and we have the scored labels and scored probabilities predicted for this set of values right here in the Excel. If you check this box, autophrenic will predict every change in the input cell without requiring you to click the Predict button. You can actually create a small predictive application using Excel and this web service. You can populate the web service with information from a small form created in a different sheet. And as the user fills in the input form, they can see the result of this prediction web service in Excel itself.
This is what I love the most about Azure. You can not only create models using the simplest possible methods but also test and deploy them using common tools such as Excel that we are already aware of. I would definitely love to know more about how you use this particular feature to deploy your models as Web services. Try it out for yourself and let me know in the comments. That concludes our session on how to deploy ML models as Web services for quick deployment and consumption. Thank you so much for joining me in this session, and I will see you in the next lecture. Until then, have a great time.
Regression Analysis
1. What is Linear Regression?
Hello and welcome. In this lecture, we are going to learn what regression is and what linear regression is. As we have seen in the four types of models, regression analysis is a statistical process for estimating the relationships among variables where the predictor is a continuous variable and the focus is on the relationship between a dependent variable and one or more independent variables. Predictors are another term for predictors. It is one of the most common methods used in machine learning in certain circumstances. It can also be used to infer causal relationships between dependent and independent variables. We also saw an example of the number of medical claims versus age, where the number of claims could be predicted based on the variable or feature age.
Now, some other examples could be predicting future sales or predicting the price of the product or service. We also looked at what is meant by a causal relationship and correlation, as well as various types of correlations such as strong positive correlation, strong negative correlation, and so on. In the case of multivariate linear regression, the equation would look something like this, where you have more than one feature predicting the value of y. Let's try to understand what simple linear regression is. In a bit more detail, we have seen this equation as well as such graphs where B0 is the intercept and B1 is the slope of the line. Y is also called a dependent variable, and x is called an independent variable. All right, let's try to solve this equation. For an example, we have this small dataset, which represents the number of hours studied by a student and the marks she scored.
We also calculated the means for x and y. Assume that the number of hours studied is x and the number of marks obtained, which is also dependent on x, is y. Next, we calculate a few more terms, such as the difference of x and x mean and the difference of y and y mean. We also square this difference, and in the last column here, we have multiplied the respective differences of x and y from their means. If you want, you can pause this video here and compute these values or recheck some of these values. However, this is only for understanding purposes after all the calculations. This is how the table would look after we have completed all the values for the x and y. Now, the formula for calculating the B-value is some of the multiplication of x and y differences from their respective means divided by the square of the x-minus-x mean. All right, at this stage, I'm not going to explain the details of how this formula has been derived, but try to understand how the values of b zero and b one are computed for a simple regression.
All right, so once we impute these figures, we get the value of B1 as 455. That's great. Now let's try to compute the value of Bzero so that we have the equation of our line and we can predict the future values of marks scored based on the number of study hours. All right, we have x and y axes here, and we have also plotted the mean of x and y. Now, the point to remember here is that every regression line always passes through the intersection of x and y means, which is nothing but this point over here.
So when we substitute the values of x and y for this particular point in this equation, we have 66.31, which is equal to b zero plus 455 multiplied by 538. I hope you are with me. When we solve this equation, we get 41.8 for b zero. So now the regression line equation for this example is y = 41.8 plus 4.55 times x. That is, the line's intercept at y = 41.8. Well, we can now predict the marks scored by any student, provided we know the number of hours she has studied. That brings us to the end of this lecture on what linear regression is. In the next lecture, we will cover ordinary list square and some more terms related to linear regression. Thank you so much for joining me in this lecture, and I will see you in the next one. Until then, have a great time.
2. Regression Analysis - Common Metrics
Hello and welcome. In the previous lecture, we learned about linear regression. What are simple regression and multivariate regression? In this lecture, we will COVID several common terms as well as one solution method for the ordinary list square. So let's start with the OLS. In this example, we have some data points plotted on the independent x-axis and dependent on the y-axis. A regression line that has an equation as a function of x is plotted like this: We have seen this in the previous lecture on how we can draw a simple linear regression line.
However, for a given value of x, let's say xi, we have an actual value of y as represented by this particular data point here, and let's call it yi. But before we were to predict the value of y based on the regression line that we have plotted, it would be represented by this data point on the regression line. This predicted value is called "Yi hat." And as you can see from this graph, the two values—actual and predicted—have a difference, and this difference is nothing but the prediction error that our regression model has committed. Similarly, the majority of the data points will have some error compared to the predicted value of y. In the ordinary list-square method, the model draws a line that has a minimum sum of squares of the error between predicted and actual values.
So among all the possible lines that can be drawn, we choose the one with the lowest cost per ordinary square of the arrows. All right, I hope that explains what the OLS method of drawing a regression line is. Even Microsoft Excel uses this method of regression. Remember, what we do is choose the line with the least cost of the ordinary square of the errors. All right. Next, let's look at some of the terms that you may encounter in regression analysis. One of them is the mean absolute error. As the name suggests, it is the mean or average of the absolute value of the error. So basically, we take the difference between the actual value and the predicted value, as the difference can be both positive as well as negative. Positives for data points above the regression line and negatives for data points below it Hence, we take the absolute value of that error and then do a sum of all these values and divide it by the number of observations.
MEA is used to measure how close the forecasts or predictions are to the eventual outcomes. The lower the MEA value, the better or more accurate the predictions in comparison to actuals. All right, the next very commonly used term is RMSE, or root means square error. Let's try to read and understand it backwards. First we calculate the error between the predicted and actual values, and then we do a square of it. This also amplifies the large errors. Then we do a sum of all the squared errors and divide it by the number of observations to get the mean of it. Finally, we take the square root of this term to arrive at the root mean square error. This is one of the most commonly used terms to understand the effectiveness of the numeric predictions. And compared to other measurements, RMSC punishes and amplifies the larger errors.
As we have seen, the square would always amplify those errors. All right, the last one from this lecture is the relative absolute error. as I suggested in the past. Try to read these terms backward to understand their meaning of it. So we have a prediction error. We consider the absolute value of the error and the sum of all such errors.
Secondly, we also do the sum of the distance of the actual values from the mean. Alright? I'll repeat: we do the sum of the distance of the actual values from the mean, which is nothing but the absolute values of the difference between the mean and actual value. All right? And the ratio of these two values is nothing but the relative absolute error. All right, that concludes this lecture on OLS and common terms used in regression. In the next lecture, let's build a model for linear regression. I'm super excited to build it with you. So I'll see you in the next class, and thank you for joining me in this one. Until then, enjoy your time.
Microsoft Certified: Azure Data Scientist Associate certification practice test questions and answers, training course, study guide are uploaded in ETE files format by real users. Study and pass Microsoft Microsoft Certified: Azure Data Scientist Associate certification exam dumps & practice test questions and answers are the best available resource to help students pass at the first attempt.