- Home
- Google Certifications
- Professional Data Engineer Professional Data Engineer on Google Cloud Platform Dumps
Pass Google Professional Data Engineer Exam in First Attempt Guaranteed!
Get 100% Latest Exam Questions, Accurate & Verified Answers to Pass the Actual Exam!
30 Days Free Updates, Instant Download!
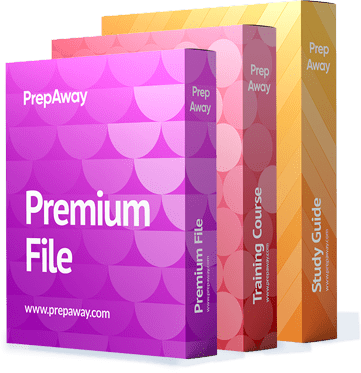
Professional Data Engineer Premium Bundle
- Premium File 319 Questions & Answers. Last update: Feb 09, 2025
- Training Course 201 Video Lectures
- Study Guide 543 Pages
Last Week Results!

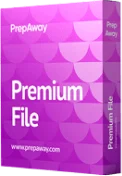
Includes question types found on the actual exam such as drag and drop, simulation, type-in and fill-in-the-blank.
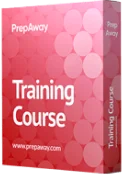
Based on real-life scenarios similar to those encountered in the exam, allowing you to learn by working with real equipment.
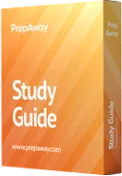
Developed by IT experts who have passed the exam in the past. Covers in-depth knowledge required for exam preparation.
All Google Professional Data Engineer certification exam dumps, study guide, training courses are Prepared by industry experts. PrepAway's ETE files povide the Professional Data Engineer Professional Data Engineer on Google Cloud Platform practice test questions and answers & exam dumps, study guide and training courses help you study and pass hassle-free!
Cloud SQL, Cloud Spanner ~ OLTP ~ RDBMS
2. Lab: Creating A Cloud SQL Instance
Cloud SQL is simply a highly available relational database running on the cloud. Let's say you are setting up a CloudSQL instance to serve live traffic on your actual website on your production deployment. What kind of underlying hard disc would you use for your cloud SQL instance? In this lecture, let's see how you would create a new Cloud SQL instance and then create a database within it all using the web console on your Google Cloud Platform dashboard.
Click on the horizontal lines on the top left and go to your side navigation bar. Choose the SQL instance, and it will take you to the Cloud SQL page. If you don't have any database instances set up, simply click on Create to create your very first Cloud SQL instance. Cloud SQL requires an instance to be set up before you can start using it, which means it is not serverless. Cloud SQL is a fully managed MySQL or PostgreSQL database service that is built on top of Google's infrastructure. It's very well suited for OLTP workloads and structured data. Based on the requirements of the application that you are setting up, you'll choose between MySQL or Post pre SQL. Do note, though, that PostgreSQL support is in beta. That means it can be changed in backward-compatible ways and does not have a strict SLA with the user. Google supports MySQL versions 5.6 or 5.7, which I'm going to use right now.
Because PostgreSQL support is in beta at this point in time, it doesn't have some of the high availability options that are available with MySQL. Google Cloud SQL databases for MySQL are available in two generations: the first generation, which is the Legacy sequel, and the second generation, which are newer versions of MySQL, which is what is recommended. The first generation of MySQL only supports older versions, namely versions 5 and 6, whereas the second generation supports newer versions. Version 5.7 is also supported. I'm going to choose the second generation of My Sorrow that's recommended, and here are all the other advantages that this offers me. Once you've made your choice, it will take you to a page where you set up the basic parameters of your SQL instance, for example, the instance ID, which I'm going to call SQLhere, and the root password for your instance. You can also choose to generate a root password, and the Web console will do it for you. You can also choose to have a no-route password set. Simply click on the check box right below the text box for the password.
Anyone who has worked with MySQL before will tell you that this is not really recommended. So I'm going to set a simple root password and then set up the location of my MySQL instance. I'll select "US Central" as my region and "US Central A" as my zone. These are the basic options. There is a list of advanced options that you can configure as well. If it's a production project, you're likely to have some customised settings that you want to work with. On Google Cloud, you can select the database version (MySQL 5.7 or 5.6) for the second generation. MySQL runs using the innovative Execution Engine. You can also choose the kind of machine on which you want to host your MySQL instance. Obviously, if you choose one with more CPUs and more memory, its processing will be a little faster. You can also configure a storage option for your MySQL. What kind of hard drive do you want your relational database stored on? The recommended value is SST, or solid state drives, because they are much faster and offer lower latency and higher QPS and data throughput. That's the default choice, and that's what is recommended.
Basic hard disks, also known as persistent discs or HDDs, perform poorly in comparison to SSDs. They are not suitable for production deployments. But if you have a test MySQL instance, you might want to choose this. You'll save on some storage costs. I've chosen a 10-gigabyte hard disk. That's the default, and that's enough for the purposes of this example and below. That is an important setting that you should generally check. As you use your Cloud SQL database in production, enable automatic storage increases. As the data within your database constantly increases, and if it exceeds your current hard disc capacity, Google will automatically add more storage. in order to accommodate increased data.
You can specify a limit up to which you want this increase to occur. This instance is going to serve live traffic. You might want to enable automatic backups and high-availability automatic backups. Back up all the data that is stored within Cloud SQL. At periodic instances, you can specify the time at which this backup should occur. You should also have binary logging enabled. That's the default setting because it allows point-in-time recovery and replication of your data. Point-in-time recovery allows you to restore or recover your data from a time in the past. If your website or web application must be highly available to your users, such as a banking application, you may require a failover replica for your Cloud SQL database.
A failover replica takes over if the original database is down for some reason. You can also choose to authorise only certain networks to access your data. This is for increased security. You can specify that only these IP addresses and no others can access My Cloud SQL database. You can also add additional Cloud SQL flags that you want to use to customise your database. There is a list of flags that are available, and you can just set them up right here. The page also allows you to configure your maintenance schedule. That's pretty self-explanatory. Let's go ahead and create this cloud SQL instance. When I tried creating it for the very first time, I got an error indicating that some of the information I'd provided was wrong. Go back and check all your settings to ensure that you haven't inadvertently added a setting that you didn't mean to. Here I set up "Add Cloud SQLflags," but I didn't really specify a flag. I need to get rid of the settings so that I can go ahead and create my database.
Give your instance a little bit of time to spin up, and there you have it: your very first cloud SQL instance running My SQL Second Generation. And then navigate to the instance and investigate all of the settings and details that are available to you. If you click on Access Control and then Users within Access Control, you can set up additional usernames and passwords that can access this particular instance. You want other users other than the root user. Here is where you add them. The Databases Link allows you to view all the databases that you have currently set up in My Sequel in the Web Console. Here are the default databases that MySQL creates whenever you create a new instance. You can also create new databases and tables within databases using the Web Console. Going back to the question at the beginning of this video, what kind of storage hard disc would you use for your cloud SQL in production mode? The answer is SSD, or solid-state State device. It provides low latency, high throughput, and is much more reliable than plain hard disks.
3. Lab: Running Commands On Cloud SQL Instance
Somewhere in this lecture, you will find the answer to this question: how does Cloud SQL allow access via the Cloud Shell command line? How can an ephemeral instance of your CloudShell get access to your MySQL database? Now that we've created a cloud SQL instance, let's actually connect to that instance using the command line.
Create database tables and perform operations on them. worked with MySQL before. It's quite likely that you use the command line to connect to your MySQL instance and then run a whole bunch of commands. You can do exactly the same thing by using Google's Cloud Shell as well. You can simply treat it as your Linux command line. As with all command-line operations using Google Cloud, we'll use the G Cloud utility.
4. Lab: Bulk Loading Data Into Cloud SQL Tables
At the end of this video, you'll learn one way in which you can bulk load data into your tables using CSV files. In this lecture, you'll see how you can create tables using SQL files stored in the cloud and bulk load data into these tables. In this lecture, we are going to run a lab from the Training Data Analyst repository. We are familiar with this repository. We worked with this before in this course here. We'd like to clone it into that repository on our cloud shell machine.
All cloud shell machines come preinstalled with git, so you do not need to install git, but you do need to clone this repository. You know that "Training Data Analyst" contains a whole bunch of code labs for big data training on the Google Cloud. Run the git clone command on your Cloud Shell command line. I already have this repository on my Cloud Shell, which is why I see this message. If you're doing this for the first time, you'll see your repository cloned onto your Cloud shell machine.
Change to lab C's directory. That's the lab that we'll work on today. All the table creation commands are present in the table underscore creation sequel file. Inside the Cloud SQL directory in LabThree A, open up table "Creation Sequel" and let's examine what commands it runs. It first creates a database called Recommendation Underscore Spark if it doesn't already exist. And within this recommendation underscore Sparkdatabase, it deletes and then creates three tables: recommendations, ratings, and accommodations. You can imagine that these are the types of rental sites that Airbnb might use. The accommodations that are available are good, whether people like them or not, as shown by the ratings and recommendations that they provide. It creates each of these tables.
Examine the columns of these tables carefully so that you understand what data is being stored within them. Within the Cloud SQL directory in Lab 38, you'll find a bunch of CSV files that hold data that are going to be loaded into these tables. You can simply check the starred CSV files in Cloud SQL, and you'll see there is a reading CSV and an accommodation CSV. I'm going to store these files, table underscore Creationdot SQL and the corresponding CSV files that contain the data, onto a bucket on Cloud Storage named lunucketSQL, and I do this using gsutil. Once these files have been copied over to cloud storage, you can run the GS utilis command on a bucket to ensure that the files have been copied over successfully. They have it here. So we are all good. Now let's switch over to the Google Cloud Platform web console.
Go to the page for SQL instances and create a brand new Cloud SQL instance. This is going to be a MySQL second generation instance, and I'm going to give it the name Rentals for the best possible performance. Do you want it close to where your bucket list is? My bucket is situated in the US. This is why I chose the United States. Central One A as the location for my Cloud SQL instance. We now want to use the MySQL command to connect to our instance from Cloud Shell. This requires that we explicitly whitelist our Cloud Shell IP for our particular instance. This we can do by going to Access Control and adding a network. Doing this will allow you to connect to your MySQL instance on Cloud SQL directly using the MySQL command rather than the G Cloud Data Connect command. Clicking on Add Network will throw up a dialogue that asks you to specify a name for this whitelist and also specify the IP address of your Cloud Shell. Remember that this IP address may change if you have to reconnect to your session, and you might have to whitelist your new IP address. In that case, if you're directly using your terminal from your local machine after having installed the Google Cloud SDK, then this problem should not occur.
Switch over to your Cloud Shell command line, and within the folder for Lab Three A, you'll find a shell script that allows you to find the IP address of your Cloud Shell. It's known as findmyresh. Simply run the script and get the IP address of your Cloud Shell for whitelisting. Put this IP address into your Web console dialogue where you want to add a network to access your SQL instance, and you're done. Your Cloud Shell IP is now whitelisted, and you can connect directly to MySQL using your Cloud Shell. On the Sequel page of your dashboard, you'll find an IP address listed against your instance.
We can use this IP address in order to connect to MySQL from our Cloud Shell command line. Let's start with your Rentals instance and set up some tables. Import the table underscore creation sequel file from your cloud storage bucket. So specify the name of your bucket and the file you want to import from within it. You can use the Browse button to see your buckets and what files you have stored within each. Find the table underscore "Creation" in the SQL file that we copied over to our cloud storage earlier. When you see a check mark next to your object, you know that the web console has validated it.
You can now click Import and import the SQL file. Google Cloud Platform will run all the Sequel commands present in that file against this rental database. In your MySQL instance, the tables will have been created. There is no data in these tables yet. Now let's bulk load data into the tables that exist within our Recommendations Park database. You can do this once again using Import Browse, the cloud storage buckets that you have available. We are now looking for CSV files. That's why we check the CSV for the format of "Import." Browse through the buckets and find the accommodation CSV that we've copied over from our local machine using GS Util. It should be within the SQL folder of our LunarUS bucket cloud platform, which will validate that the CSV file exists, and once you get a green checkmark, you can go ahead and specify the database where you want the CSV to be loaded and the table within it. The database is Recommendation underscore Spark, and the table is Accommodation. Click on Import, and all the data within the CSV file will be bulk imported into that table. If you notice the usage graph for your rental SQL instance, you'll see some activity. This is because of the bulk import of data and the creation of tables that we just carried out.
Click on the Databases link, and you'll find that the Recommendations bar is now present within your MySQL databases. For this instance, you can follow the exact same process to bulk load CSV data into other tables that you have. For example, the Ratings table can get data from the Ratings CSV. Make sure you specify the correct file from your cloud storage, the correct database, and the correct tablet that you want this data to be loaded into. Remember, though, that the schema of your CSV file must match the schema of the tables where you're loading data. Otherwise, the import will fail. Let's switch over to the Cloud Shell command line and connect to our MySQL instance to see whether the data has been loaded successfully. Here we are directly using the MySQL command, which is why we specify the host, the user, and the password in the format that you see.
This is a very standard MySQL connection operation, and you're probably familiar with it because we whitelisted our Cloud Shell IP address earlier. This connection just works. Otherwise, you'll have to use Google Cloud Platform Connect to connect to your SQL. In the host parameter, specify the IP address of your SQL instance that you got from the web console, and in the password parameters, specify your username and password. Specify the password for your root user. In my case, it was just a test password. You should be able to connect to your SQL instance just fine. Use the recommendation underscore Spark DB, and let's run some queries on our tables. Accommodations and ratings have data within them. A simple select query on Accommodations should show us that the data from the CSV file has been loaded just fine. In this lecture, you saw how you could create tables in your cloud SQL instance using commands stored in a file that is located in your cloud storage. You can also bulk load data in the same way that your CSV files can live in cloud storage, and you simply import it into your database.
5. Cloud Spanner
What is horizontal scaling? This is a question that I'd like you to think about. What is horizontal scaling, specifically in the context of the Google Cloud Platform, and what relational database product offers it? Please think about this. Keep this at the back of your mind as you go through this material. We will come back to the answer at the end of this video.
Let's keep going down our list of use cases. And next, let's talk about cloud spanner. Cloud Spanner is another option for transaction processing that's available under the Google Cloud suite. This is in some sense an alternative to Cloud SQL, as we have already referred to it on multiple occasions. It is a Google proprietary technology, and it offers horizontal scaling. This means that if you have bigger datasets to support, all you really need to do is add more node instances. In my opinion, cloud computing is a really interesting technology. Just the insights into what's going on under the hood make studying this topic worthwhile. Use Cloud Spanner when you need really high availability or when you need really strong consistency.
As you shall see, Cloud Spanner has asset-plus-plus transaction support. In general, anytime you have transactional reads and writes, especially writes, you've got to use either Cloud SQL or Cloud Spanner. If you need high availability and strong consistency, then go with Cloud Spanner. It bears repeating: do not use CloudSpanner unless you need relational database support. It's kind of obvious, but it's worth keeping in mind if your data does not lend itself well to the relational data model. If it's not well structured, do not use an RDBMS. We also should not use Cloud Spanner if we explicitly want to make use of open source technologies. because, as discussed, Cloud Spanner is Google proprietary. And lastly, remember that Cloud Spanner makes really strong guarantees about consistency. More on that in a bit. So don't use it if you don't require the strongest levels of consistency in your application. We are now getting to the really interesting bits about the data model in Cloud Spanner. Superficially, this seems very similar to how cloud SQL databases contain tables, just as usual, and those tables look relational.
They have rows, columns, and strongly typed schemas, just like Cloud SQL tables. But it turns out that there is a lot more going on under the hood. So let's examine some of the internal implementation details of Cloud Spanner. Let's start with simple relational data. As we had previously discussed, relational data is arranged in tables. Tables have rows and columns. The columns, the number, the types, and the names of those columns are clearly defined up front. And let's take a really simple sample. We have three relatives here. The first of these contains student data. That's two columns: student ID and student name. The second contains course data. This is the course ID. and course name. And then the third contains a bunch of information about students and their grades. Think of it as transcript information. So the columns in this last relationship are studentID, student name, course ID, term, and grade.
As you can see, these are a concatenation of the columns from the student and course tables. The interesting difference between cloud spanner and a traditional Rtbm is that the underlying representation of this data can be changed based on usage patterns and query patterns. This is not something that would really be possible in a traditional RDBMS. Let's say, for instance, that we usually query student and course grades together, and that's because our most common query is effectively to get the transcript of a student. What cloud spinning allows us to do is specify a parent-child relationship between these two tables. So if we specify that the course transcript data is, in some sense, a child of the student data, the representation of this data will be interleaved. Every row from the student table will be followed by all of the rows from the child transcript table that have the same primary key value, which is the student ID. And that's why we have the rows corresponding to the student Jane Doe all clumped together. If you're thinking that this physical representation looks a little bit like each base because we have all of the key values sequentially sorted, well, you're right on the money.
CloudSpanner's data representation has some intriguing similarities with HBase. Let's come back to the parent-child relationship. This is something that one can specify between tables up to seven levels of nesting, and these cause the physical locations of those tables to be interleaved. Just as we saw, this causes access to be very fast because now if you want to query all of the data for a particular row ID, let's say for student ID equal to one, all of those rows are going to appear together on disk. Once again, let's be certain we understand what just happened. Because we often query data for students and their grades together, we made the grades in the transcript stable. Data locality was then enforced between two seemingly independent tables using a child of the student's table. A requirement of CloudSpanner is that every table must have a primary key. This once again is rather H-base-like or big table-like in its thought process.
Now, by declaring that one table is a child of another, we are in effect causing CloudSpender to prefix the parent's primary key onto the primary key of the child. And once again, this is distinctly H-based in its philosophy. Once we've tacked on the primary key of the parent onto the primary key of the child, interleaving can then be implemented. Rows will be sorted and then stored in the sorted order of those primary key values. Child rows are going to be inserted between parent rows, which is where the term for this way of doing things arises because, of course, the tables are really literally interleaved.
Now, all values that relate to a particular value of the parent primary key can be easily picked off by just using a scan. And this sequential scan, once again, is exactly the kind of operation that one associates with HBase or BigTable. Let's come back to the question we posed at the start of this video. The question was, "What is horizontal scaling?" Hopefully, we've now understood that horizontal scaling refers to an increase in the capacity of our system or our software merely by adding additional machines.
So by doing more of the same without increasing the resources, the CPU, or the memory of the existing machines, That is vertical scaling. Cloud spanner is a great example of a tool that offers horizontal scaling, and it does so in the typical Google cloud platform way. It adds server capacity on the fly based on how much your application requires. The contrast to this would be vertical scaling, where we would move towards a more monolithic database server kind of model like Trent relational databases, where we would need to add additional memory or disc space to a single machine. Horizontal scaling corresponds loosely to distributed computing. Vertical scaling corresponds to monolithic computing.
Google Professional Data Engineer practice test questions and answers, training course, study guide are uploaded in ETE Files format by real users. Study and Pass Professional Data Engineer Professional Data Engineer on Google Cloud Platform certification exam dumps & practice test questions and answers are to help students.
Exam Comments * The most recent comment are on top
Purchase Professional Data Engineer Exam Training Products Individually
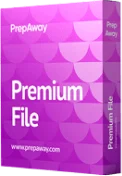
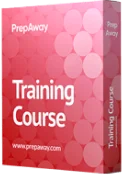
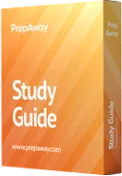
Why customers love us?
What do our customers say?
The resources provided for the Google certification exam were exceptional. The exam dumps and video courses offered clear and concise explanations of each topic. I felt thoroughly prepared for the Professional Data Engineer test and passed with ease.
Studying for the Google certification exam was a breeze with the comprehensive materials from this site. The detailed study guides and accurate exam dumps helped me understand every concept. I aced the Professional Data Engineer exam on my first try!
I was impressed with the quality of the Professional Data Engineer preparation materials for the Google certification exam. The video courses were engaging, and the study guides covered all the essential topics. These resources made a significant difference in my study routine and overall performance. I went into the exam feeling confident and well-prepared.
The Professional Data Engineer materials for the Google certification exam were invaluable. They provided detailed, concise explanations for each topic, helping me grasp the entire syllabus. After studying with these resources, I was able to tackle the final test questions confidently and successfully.
Thanks to the comprehensive study guides and video courses, I aced the Professional Data Engineer exam. The exam dumps were spot on and helped me understand the types of questions to expect. The certification exam was much less intimidating thanks to their excellent prep materials. So, I highly recommend their services for anyone preparing for this certification exam.
Achieving my Google certification was a seamless experience. The detailed study guide and practice questions ensured I was fully prepared for Professional Data Engineer. The customer support was responsive and helpful throughout my journey. Highly recommend their services for anyone preparing for their certification test.
I couldn't be happier with my certification results! The study materials were comprehensive and easy to understand, making my preparation for the Professional Data Engineer stress-free. Using these resources, I was able to pass my exam on the first attempt. They are a must-have for anyone serious about advancing their career.
The practice exams were incredibly helpful in familiarizing me with the actual test format. I felt confident and well-prepared going into my Professional Data Engineer certification exam. The support and guidance provided were top-notch. I couldn't have obtained my Google certification without these amazing tools!
The materials provided for the Professional Data Engineer were comprehensive and very well-structured. The practice tests were particularly useful in building my confidence and understanding the exam format. After using these materials, I felt well-prepared and was able to solve all the questions on the final test with ease. Passing the certification exam was a huge relief! I feel much more competent in my role. Thank you!
The certification prep was excellent. The content was up-to-date and aligned perfectly with the exam requirements. I appreciated the clear explanations and real-world examples that made complex topics easier to grasp. I passed Professional Data Engineer successfully. It was a game-changer for my career in IT!