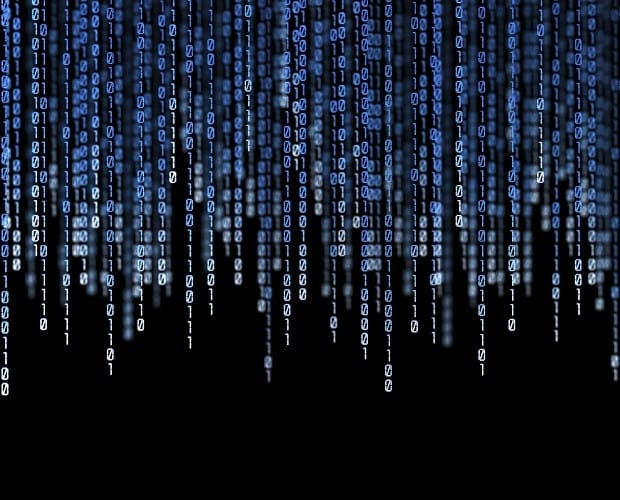
Professional Data Engineer: Professional Data Engineer on Google Cloud Platform Certification Video Training Course
The complete solution to prepare for for your exam with Professional Data Engineer: Professional Data Engineer on Google Cloud Platform certification video training course. The Professional Data Engineer: Professional Data Engineer on Google Cloud Platform certification video training course contains a complete set of videos that will provide you with thorough knowledge to understand the key concepts. Top notch prep including Google Professional Data Engineer exam dumps, study guide & practice test questions and answers.
Professional Data Engineer: Professional Data Engineer on Google Cloud Platform Certification Video Training Course Exam Curriculum
You, This Course and Us
-
1. You, This Course and Us
Introduction
-
1. Theory, Practice and Tests
-
2. Lab: Setting Up A GCP Account
-
3. Lab: Using The Cloud Shell
Compute
-
1. Compute Options
-
2. Google Compute Engine (GCE)
-
3. Lab: Creating a VM Instance
-
4. More GCE
-
5. Lab: Editing a VM Instance
-
6. Lab: Creating a VM Instance Using The Command Line
-
7. Lab: Creating And Attaching A Persistent Disk
-
8. Google Container Engine - Kubernetes (GKE)
-
9. More GKE
-
10. Lab: Creating A Kubernetes Cluster And Deploying A Wordpress Container
-
11. App Engine
-
12. Contrasting App Engine, Compute Engine and Container Engine
-
13. Lab: Deploy And Run An App Engine App
Storage
-
1. Storage Options
-
2. Quick Take
-
3. Cloud Storage
-
4. Lab: Working With Cloud Storage Buckets
-
5. Lab: Bucket And Object Permissions
-
6. Lab: Life cycle Management On Buckets
-
7. Lab: Running A Program On a VM Instance And Storing Results on Cloud Storage
-
8. Transfer Service
-
9. Lab: Migrating Data Using The Transfer Service
-
10. Lab: Cloud Storage ACLs and API access with Service Account
-
11. Lab: Cloud Storage Customer-Supplied Encryption Keys and Life-Cycle Management
-
12. Lab: Cloud Storage Versioning, Directory Sync
Cloud SQL, Cloud Spanner ~ OLTP ~ RDBMS
-
1. Cloud SQL
-
2. Lab: Creating A Cloud SQL Instance
-
3. Lab: Running Commands On Cloud SQL Instance
-
4. Lab: Bulk Loading Data Into Cloud SQL Tables
-
5. Cloud Spanner
-
6. More Cloud Spanner
-
7. Lab: Working With Cloud Spanner
BigTable ~ HBase = Columnar Store
-
1. BigTable Intro
-
2. Columnar Store
-
3. Denormalised
-
4. Column Families
-
5. BigTable Performance
-
6. Lab: BigTable demo
Datastore ~ Document Database
-
1. Datastore
-
2. Lab: Datastore demo
BigQuery ~ Hive ~ OLAP
-
1. BigQuery Intro
-
2. BigQuery Advanced
-
3. Lab: Loading CSV Data Into Big Query
-
4. Lab: Running Queries On Big Query
-
5. Lab: Loading JSON Data With Nested Tables
-
6. Lab: Public Datasets In Big Query
-
7. Lab: Using Big Query Via The Command Line
-
8. Lab: Aggregations And Conditionals In Aggregations
-
9. Lab: Subqueries And Joins
-
10. Lab: Regular Expressions In Legacy SQL
-
11. Lab: Using The With Statement For SubQueries
Dataflow ~ Apache Beam
-
1. Data Flow Intro
-
2. Apache Beam
-
3. Lab: Running A Python Data flow Program
-
4. Lab: Running A Java Data flow Program
-
5. Lab: Implementing Word Count In Dataflow Java
-
6. Lab: Executing The Word Count Dataflow
-
7. Lab: Executing MapReduce In Dataflow In Python
-
8. Lab: Executing MapReduce In Dataflow In Java
-
9. Lab: Dataflow With Big Query As Source And Side Inputs
-
10. Lab: Dataflow With Big Query As Source And Side Inputs 2
Dataproc ~ Managed Hadoop
-
1. Data Proc
-
2. Lab: Creating And Managing A Dataproc Cluster
-
3. Lab: Creating A Firewall Rule To Access Dataproc
-
4. Lab: Running A PySpark Job On Dataproc
-
5. Lab: Running The PySpark REPL Shell And Pig Scripts On Dataproc
-
6. Lab: Submitting A Spark Jar To Dataproc
-
7. Lab: Working With Dataproc Using The GCloud CLI
Pub/Sub for Streaming
-
1. Pub Sub
-
2. Lab: Working With Pubsub On The Command Line
-
3. Lab: Working With PubSub Using The Web Console
-
4. Lab: Setting Up A Pubsub Publisher Using The Python Library
-
5. Lab: Setting Up A Pubsub Subscriber Using The Python Library
-
6. Lab: Publishing Streaming Data Into Pubsub
-
7. Lab: Reading Streaming Data From PubSub And Writing To BigQuery
-
8. Lab: Executing A Pipeline To Read Streaming Data And Write To BigQuery
-
9. Lab: Pubsub Source BigQuery Sink
Datalab ~ Jupyter
-
1. Data Lab
-
2. Lab: Creating And Working On A Datalab Instance
-
3. Lab: Importing And Exporting Data Using Datalab
-
4. Lab: Using The Charting API In Datalab
TensorFlow and Machine Learning
-
1. Introducing Machine Learning
-
2. Representation Learning
-
3. NN Introduced
-
4. Introducing TF
-
5. Lab: Simple Math Operations
-
6. Computation Graph
-
7. Tensors
-
8. Lab: Tensors
-
9. Linear Regression Intro
-
10. Placeholders and Variables
-
11. Lab: Placeholders
-
12. Lab: Variables
-
13. Lab: Linear Regression with Made-up Data
-
14. Image Processing
-
15. Images As Tensors
-
16. Lab: Reading and Working with Images
-
17. Lab: Image Transformations
-
18. Introducing MNIST
-
19. K-Nearest Neigbors
-
20. One-hot Notation and L1 Distance
-
21. Steps in the K-Nearest-Neighbors Implementation
-
22. Lab: K-Nearest-Neighbors
-
23. Learning Algorithm
-
24. Individual Neuron
-
25. Learning Regression
-
26. Learning XOR
-
27. XOR Trained
Regression in TensorFlow
-
1. Lab: Access Data from Yahoo Finance
-
2. Non TensorFlow Regression
-
3. Lab: Linear Regression - Setting Up a Baseline
-
4. Gradient Descent
-
5. Lab: Linear Regression
-
6. Lab: Multiple Regression in TensorFlow
-
7. Logistic Regression Introduced
-
8. Linear Classification
-
9. Lab: Logistic Regression - Setting Up a Baseline
-
10. Logit
-
11. Softmax
-
12. Argmax
-
13. Lab: Logistic Regression
-
14. Estimators
-
15. Lab: Linear Regression using Estimators
-
16. Lab: Logistic Regression using Estimators
Vision, Translate, NLP and Speech: Trained ML APIs
-
1. Lab: Taxicab Prediction - Setting up the dataset
-
2. Lab: Taxicab Prediction - Training and Running the model
-
3. Lab: The Vision, Translate, NLP and Speech API
-
4. Lab: The Vision API for Label and Landmark Detection
Virtual Machines and Images
-
1. Live Migration
-
2. Machine Types and Billing
-
3. Sustained Use and Committed Use Discounts
-
4. Rightsizing Recommendations
-
5. RAM Disk
-
6. Images
-
7. Startup Scripts And Baked Images
VPCs and Interconnecting Networks
-
1. VPCs And Subnets
-
2. Global VPCs, Regional Subnets
-
3. IP Addresses
-
4. Lab: Working with Static IP Addresses
-
5. Routes
-
6. Firewall Rules
-
7. Lab: Working with Firewalls
-
8. Lab: Working with Auto Mode and Custom Mode Networks
-
9. Lab: Bastion Host
-
10. Cloud VPN
-
11. Lab: Working with Cloud VPN
-
12. Cloud Router
-
13. Lab: Using Cloud Routers for Dynamic Routing
-
14. Dedicated Interconnect Direct and Carrier Peering
-
15. Shared VPCs
-
16. Lab: Shared VPCs
-
17. VPC Network Peering
-
18. Lab: VPC Peering
-
19. Cloud DNS And Legacy Networks
Managed Instance Groups and Load Balancing
-
1. Managed and Unmanaged Instance Groups
-
2. Types of Load Balancing
-
3. Overview of HTTP(S) Load Balancing
-
4. Forwarding Rules Target Proxy and Url Maps
-
5. Backend Service and Backends
-
6. Load Distribution and Firewall Rules
-
7. Lab: HTTP(S) Load Balancing
-
8. Lab: Content Based Load Balancing
-
9. SSL Proxy and TCP Proxy Load Balancing
-
10. Lab: SSL Proxy Load Balancing
-
11. Network Load Balancing
-
12. Internal Load Balancing
-
13. Autoscalers
-
14. Lab: Autoscaling with Managed Instance Groups
Ops and Security
-
1. StackDriver
-
2. StackDriver Logging
-
3. Lab: Stackdriver Resource Monitoring
-
4. Lab: Stackdriver Error Reporting and Debugging
-
5. Cloud Deployment Manager
-
6. Lab: Using Deployment Manager
-
7. Lab: Deployment Manager and Stackdriver
-
8. Cloud Endpoints
-
9. Cloud IAM: User accounts, Service accounts, API Credentials
-
10. Cloud IAM: Roles, Identity-Aware Proxy, Best Practices
-
11. Lab: Cloud IAM
-
12. Data Protection
Appendix: Hadoop Ecosystem
-
1. Introducing the Hadoop Ecosystem
-
2. Hadoop
-
3. HDFS
-
4. MapReduce
-
5. Yarn
-
6. Hive
-
7. Hive vs. RDBMS
-
8. HQL vs. SQL
-
9. OLAP in Hive
-
10. Windowing Hive
-
11. Pig
-
12. More Pig
-
13. Spark
-
14. More Spark
-
15. Streams Intro
-
16. Microbatches
-
17. Window Types
About Professional Data Engineer: Professional Data Engineer on Google Cloud Platform Certification Video Training Course
Professional Data Engineer: Professional Data Engineer on Google Cloud Platform certification video training course by prepaway along with practice test questions and answers, study guide and exam dumps provides the ultimate training package to help you pass.
Prepaway's Professional Data Engineer: Professional Data Engineer on Google Cloud Platform video training course for passing certification exams is the only solution which you need.
Pass Google Professional Data Engineer Exam in First Attempt Guaranteed!
Get 100% Latest Exam Questions, Accurate & Verified Answers As Seen in the Actual Exam!
30 Days Free Updates, Instant Download!
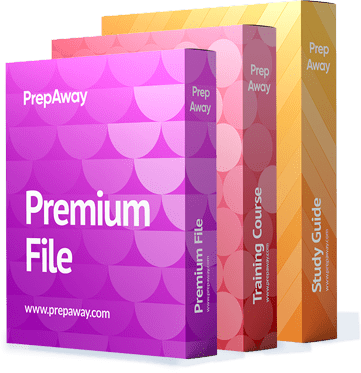
Professional Data Engineer Premium Bundle
- Premium File 319 Questions & Answers. Last update: Jul 01, 2025
- Training Course 201 Video Lectures
- Study Guide 543 Pages
Student Feedback
Comments * The most recent comment are at the top
Can View Online Video Courses
Please fill out your email address below in order to view Online Courses.
Registration is Free and Easy, You Simply need to provide an email address.
- Trusted By 1.2M IT Certification Candidates Every Month
- Hundreds Hours of Videos
- Instant download After Registration
A confirmation link will be sent to this email address to verify your login.
Please Log In to view Online Course
Registration is free and easy - just provide your E-mail address.
Click Here to Register